Three Golden Rules...
…of entropy source (thus TRNG) design.
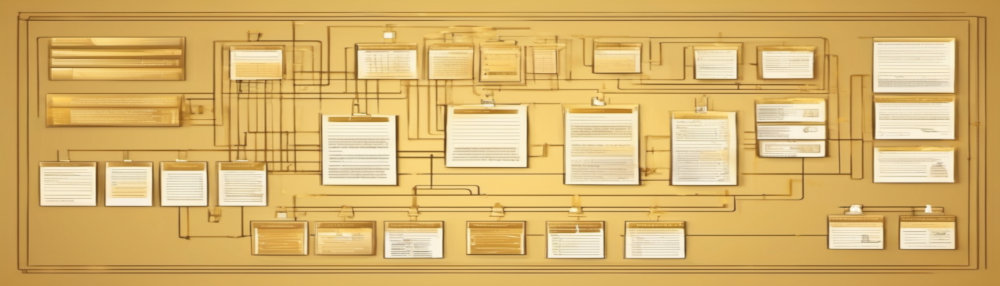
Walter White, most fittingly a.k.a. “Heisenberg” from Breaking Bad said “There is gold in the streets. Just waiting for someone to come and scoop it up.” Which can be interpreted as a reimagining of the 2nd law of thermodynamics, with entropy all around us, just waiting to be collected. Which is achieved by adhering to these three golden rules:-
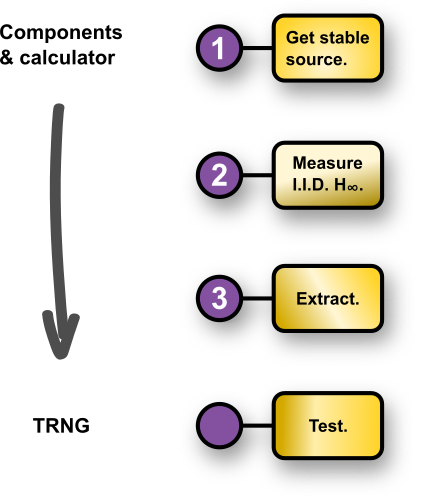
Follow these simple golden rules, and you’ll find that designing and building your own entropy source and TRNG isn’t all that difficult. Certainly not as difficult as They say. But They would say that, wouldn’t they? So explore each of the three (?) steps above↑ via the main menu← to read more details, and discover the three corresponding golden formulae.
Just ask us if you have any questions/doubts/insecurities.
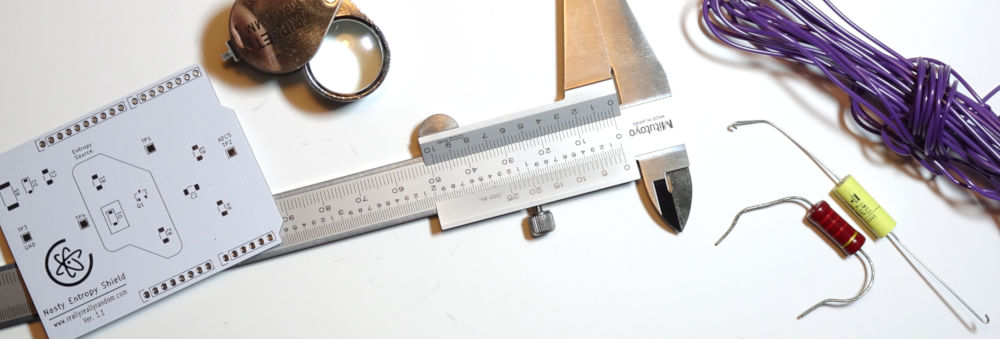